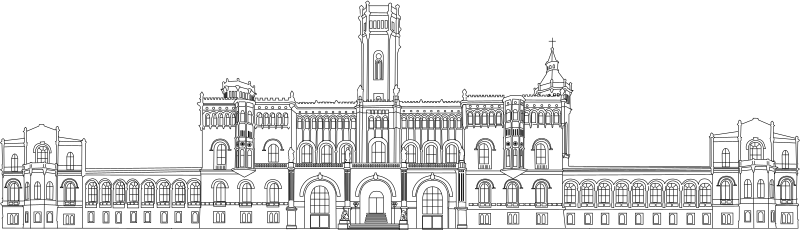
Artificial intelligence (AI) technologies are the driving force behind digitization. Due to their enormous social relevance, a responsible use of AI is of particular importance. The research and application of responsible AI is a very young discipline and requires the bundling of research activities from different disciplines in order to design and apply AI systems in a reliable, transparent, secure and legally acceptable way.
The PhD program addresses the interdisciplinary research challenges within the framework of 14 transdisciplinary doctoral programs. Organized in four clusters, fellows explore the most pressing research questions in the areas of quality, liability, interpretability, responsible use of information and the application of AI. The innovative, goal-oriented and internationally oriented supervision concept and the experienced PI team support the fellows in excellent research.
Projects
Project Overview
Project Details
Classic quality assurance procedures are based on a complete functional specification of the desired (and unwanted) system behavior. However, these methods could not be used for components whose functionality is gained through an AI method from the area of machine learning. If we had a complete functional specification of behavior, we would implement these components using a conventional process and quality assurance using established processes. For AI components, we therefore need quality assurance procedures which do not check the complete functional correctness, but which deal with the basic correctness in terms of operational safety. In this PhD project, the concept of a minimum safety specification for AI components is being developed, which determines a minimal environment for the safe functioning of an AI component (safety envelope). Building on this, test procedures are developed that generate a sufficient number of test cases for AI components in order to guarantee the correct functioning within the safety envelope up to an acceptable residual risk. Automatic driving is used as an application example, where the safety envelope for example may refer to freedom from collisions.
Principal Investigators | Prof. Dr. Ina Schaefer and Prof. Dr. Fabian Schmieder |
If a decision of an AI leads to a damaging event (e.g. personal injury or damage to property), e.g. an autonomously driving vehicle injures or even kills a pedestrian, the question immediately arises as to who might be obliged to compensate for the damage caused and to what extent. The answer is particularly important for the manufacturers of autonomously driving vehicles in determining the liability risk. The liability law for AI proceedings is therefore also the subject of controversial discussions within the legal profession, which will be the starting point of this doctoral thesis. In addition to the classification of AI in the existing liability regime, the work will mainly focus on developing a proposal for a liability concept for AI, which systematically links the different addressees of liability claims (e.g. manufacturers, operators, operators) and the type of liability in question (strict liability, strict liability), the burden of proof, the standard of liability and possible exculpation possibilities. The work offers an interdisciplinary starting point for the “operational safety of intelligent components” and the test procedures to be developed there, which - under legal conditions yet to be determined - could be taken into account under liability law, e.g. as an exculpatory possibility of the manufacturer.
Principal Investigators | Prof. Dr. Fabian Schmieder and Prof. Dr. Ina Schaefer |
With the increasing use of AI algorithms for automated decision support, the question “How objective are proposals / decisions of the used AI” becomes more and more important. The starting point is the question of “representativeness of data”, a classical topic in statistics, whose approaches (e.g. randomized / representative data samples) can, however, only partially be applied to the data selection for AI algorithms. The aim is to cover all relevant classes / cases / situations by means of a sufficiently large and representative data set, and to correctly map the distinction between the different classes. The former is complex because “all” data will never be available. Nevertheless, under certain assumptions, statements can and should of course be made about the representativeness of data used for modeling. The second aspect is even more complex, because here the interactions between data and model assumptions become relevant. Relevant in this context are, among others, approaches of “Adversarial AI”, because the learned models depend on boundary conditions of the AI algorithms, especially form and complexity of the used functions, and therefore the class boundaries of the learned models often do not reflect reality correctly. In addition to the theoretical work, this thesis will focus on two use cases with connections to the other clusters (automatic driving and applicant selection).
Principal Investigators | Prof. Dr. Wolfgang Nejdl and Prof. Dr. Felipe Temming |
Dealing responsibly with AI in the digital society is also affecting and changing companies’ operating processes. With respect to labor law, questions of explicability, fairness, transparency, data security and liability will rise while AI selects appropriate candidates. In fact, the trend can be observed that large companies in particular, are increasingly relying on AI. The use of modern psychometric tests is highly controversial due to their potentiality of automated analysis as regards the candidate’s personality. The focus of the legal issues is on the relevant decision making process as regards the applicant (AI or person) as well as on questions of liability and risk of litigation. Similarly, the question of possible disclosure of the algorithm is raised. A systematic, simultaneously comparative legal analysis of this topic is quite challenging, let alone in a monograph. Interdisciplinary links can be established to computer science, sociology and psychology programs. The PhD-project also offers the possibility for empirical studies and thus a hands-on perspective, e.g. by cooperating with companies which utilize AI in application processes, or by getting in touch with public authorities, such as the Federal Anti-Discrimination Agency (FADA).
Principal Investigators | Prof. Dr. Felipe Temming and Prof. Dr. Wolfgang Nejdl |
Human decision-making processes are not comprehensible. Knowledge of the motivation leading to a decision is reserved for the individual, because neither (according to the current state of knowledge) can the functions of the human brain be evaluated in this way, nor would this be compatible with human dignity. Since an AI cannot invoke human dignity, the question arises whether and how this principle could also apply to the decision-making process of an AI, or vice versa, which is legally required with regard to the traceability of AI decision-making processes. In addition, it must be examined whether the right to explain AI decisions, which is derived from data protection law, is sufficiently taken into account de lege lata, and how and where it should be anchored de lege ferenda, if gaps in protection can be identified.
Principal Investigators | Prof. Dr. Tina Kruegel and Prof. Dr. Wolf-Tilo Balke |
Over the last 15 years, numerous research projects have been conducted on the semantification of the World Wide Web, the so-called Semantic Web. While the actual vision is still lacking, the research has led to a meaningful and useful standardization for the representation and semantic labeling of content and a certain degree of semantic linking (W3C standards: RDF, OWL. etc.). This PhD project will investigate how current information extraction techniques (NER, OpenIE, etc.) can be successfully used together with existing knowledge from Linked Open Data (LOD) sources and crowdsourcing-based techniques to verify new knowledge, or at least assess its plausibility. Starting from AI systems' decisions, this project will develop methods to create a logically coherent chain of justification from knowledge fragments already existing on the Web. This means that a second intelligent system controls the first AI trying to offer a comprehensible justification on the output of the original system, or in the negative case to show the lack of plausibility and even point to discrimination.
Principal Investigators | Prof. Dr. Wolf-Tilo Balke and Prof. Dr. Astrid Nieße |
Distributed artificial intelligence (DAI) is used, among other application areas, for cooperative problem solving as part of distributed heuristics for optimization. While the optimization process of these algorithms is often not deterministic, a traceability of the solution process would be possible in principle by appropriately tracking convergence conditions. However, the amount of data and the representation of the solution process is problematic. A possible approach to overcome these issues is the determination of decision anchors, which are recorded exemplarily. On the basis of these decision anchors, which are ideally also distributed (e.g. by means of distributed transaction systems), visualizations can be developed that answer the need for explanation and allow algorithmic traceability. This doctoral project is therefore dedicated to the derivation and visualization of decision anchors for cooperative algorithms with distributed AI.
Principal Investigators | Prof. Dr. Astrid Nieße and Prof. Dr. Tina Kruegel |
The aim of this PhD project is to present the concepts studied (points of view, claims, facts, entities, characteristics) as part of a rich graph of knowledge that allows a qualitative evaluation and comparison of statements and their individual trustworthiness in a given context as well as their development over time. In addition to the representation of the temporal development of entities, topics, statements and their relationships, the efficient representation of controversy, bias, information quality and representative features is addressed with the aim of facilitating an efficient, reason-based query and verification of statements. Wherever possible, established vocabularies such as PROV-DM, schema.org or SIOC are used to capture contextual features such as provenance or events on the web. While the analysis of connectivity and relationship in highly networked knowledge graphs is complex and computationally intensive, connectivity metrics are considered explicit relationships. To enable efficient querying and retrieval, approaches for dimension reduction and feature aggregation are used and developed based on the queries defined in the pilot studies. The latter forms the basis for the evaluation of the knowledge graphs obtained with regard to their ability to efficiently answer the formulated questions and information needs.
Principal Investigators | Prof. Dr. Sören Auer and Prof. Dr. Ralph Ewerth |
Goal of the project is to develop methods to learn and to use word embeddings (learned semantic representations of words) that are able to deal with a bias in the training data. First, bias in word embeddings has to be defined and methods have to be developed to detect various types of bias in word embeddings. Subsequently we aim to make biases visible, e.g. by transforming the latent dimensions of the word embeddings into interpretable dimensions, as was done by Rothe et al. (2016) and Hollis and Westbury (2016). Thus reasons for the classification of a word or for similarity between words can be made transparent. Finally, ways to debias word embeddings have to be found and it has to be investigated whether approaches like those of Bolukbasi et al. (2016) und Zhao et al. (2018) to remove gender bias carry over to other types of bias like those for age, skin color, but also biases for genre or text types. Applications, like the detection of offensive language, can benefit from the findings that will help to reduce the probability to make wrong associations and to draw wrong conclusions.
Principal Investigators | Prof. Dr. Christian Wartena and Prof. Dr. Eirini Ntoutsi |
When analyzing multimodal news, several aspects have to be considered: the function of the image for a text (illustration, decoration, presentation of a concrete message aspect), the image content and its textual reference (i.e. who or what is to be seen at which event?), intended emotional message, as well as the process of image creation, i.e. is it an original, an adaptation or a composition. The state of the art shows that there is little work to date on the (semi-)automatic recognition of distorted multimodal news or fake news.
The PhD project systematically models multimodal aspects and investigates how distortions and fake news can manifest themselves in multimodal news in terms of form and content. One focus is to automatically detect formal relationships between image content and text and to develop AI methods for this purpose. In this respect, it seems to be promising to explore the potential of Generative Adversarial Networks.
Ultimately, an interactive analytics software is to be developed that supports people in evaluating the plausibility of multimodal messages. System hints can refer, for example, to where a photo was probably taken, or whether there are hints of image composition or manipulation.
Principal Investigators | Prof. Dr. Ralph Ewerth and Prof. Dr. Christian Wartena |
The aim of this PhD project is to investigate distortion in social networks such as Twitter and its effect on opinion formation and change of opinion. This question is a central challenge for many applications, from online surveys to the placement of advertising. UGC (User Generated Content) is very subjective and often reflects a wide variety of distortions and prejudices. Furthermore, in social networks, users are offered or denied content by AI algorithms based on user information such as their location, click behavior and search history. The result is isolation in cultural or ideological bubbles (filter bubble). In principle, service providers have the possibility to prefer or suppress certain opinions, e.g. in politics, economics or on migration issues. Research into UGC is also affected by bias: Only about 1% of all tweets on Twitter are available for UGC research on Twitter. Studies show that bias also occurs here. The identification of bias in opinion formation and change of opinion is highly complex. Therefore, this PhD project investigates the effects of bias on opinion formation and change of opinion. The main focus will be on how opinions are formed and how users' opinions change over time.
Principal Investigators | Prof. Dr. Eirini Ntoutsi and Prof. Dr. Christian Wartena |
Security aspects play a central role in the responsible use of AI. Attacks such as adversarial examples, evasion or mimicry attacks, membership or property inference, model inversion or stealing, poisoning or backdoors can lead to malicious and deliberate misclassifications, compromise the privacy of confidential or personal data from a learned model or manipulate training data or models before they are used. When developing AI systems, software engineers must be aware of these attacks. Known security problems in AI systems show that software engineers are often overstrained at this point.
Therefore, in the context of this PhD thesis we will investigate the causes of current problems and then explore new mechanisms and supporting tools and APIs for AI development that focus on IT security and usability for software developers. Such a developer-centered approach to AI security will allow a much more responsible use of AI in the future.
Principal Investigators | Prof. Dr. Sascha Fahl and Prof. Dr. Stefanie Büchner |
In the context of the Wikimedia Foundation, many projects on various topics have been developed in addition to Wikipedia. These include Wikimedia Commons (images, video, and audio) and Wikidata (facts). Over the last few years, numerous suggestion tools have been developed in this regard, which should make it easier for the active community to create, edit, and annotate content. Increasingly, AI tools are being applied in this area, which provide users with recommendations for curating, editing and annotating content, thereby influencing their decisions.
How does the community change when AI assists or takes the lead in various tasks? What dynamics arise in the interplay between AI-supported proposals, editing environments with the presence of version history and, in general, provenance information on metadata? How do suggestion functions in general affect the work of volunteers with often very extensive specialist knowledge in their field, including possible effects on intrinsic motivation and incentive systems such as finely granular authorship information? What are the ethical considerations when machines suddenly take over central tasks in community-centered activities?
Principal Investigators | Prof. Dr. Ina Blümel and Prof. Dr. Stefanie Büchner |
The development of responsible AI is also challenging from a sociological point of view: this cluster is taking advantage of the unique opportunity for recursive research. It opens its subprojects to a sociological-ethnographically oriented doctoral project that empirically observes how responsible AI is inscribed and translated from a social value into concrete technology in research and practice.
Three questions will guide the project: Which common and different understandings of responsible AI emerge in the course of the project and how stable and dynamic are these? What different social logics drive these understandings? How is responsibility constructed and distributed in the development process between technology and human actors? The exclusive field access to selected projects of the PhD program in different clusters opens up unique opportunities to gain empirical insights into the future central processes of making technologies and research accountable.
Principal Investigators | Prof. Dr. Stefanie Büchner, Prof. Dr. Ina Blümel and Prof. Dr. Sascha Fahl |
Partners
The L3S is a joint central institution of the Leibniz University of Hannover and the Technical University of Braunschweig with the goal of interdisciplinary research in the field of Web Science and Digital Transformation and plays a leading role in these areas both nationally and internationally. It bundles the necessary core competencies from the fields of computer science, law and sociology to research intelligent, reliable and responsible systems. Through research, development and consulting, the L3S plays a decisive role in shaping digital transformation, especially in the areas of mobility, health, production and education.
Prof. Dr. Sören Auer, Prof. Dr. Wolf-Tilo Balke, Prof. Dr. Stefanie Büchner, Prof. Dr. Ralph Ewerth, Prof. Dr. Sascha Fahl, Prof. Dr. Tina Kruegel, Prof. Dr. Wolfgang Nejdl, Prof. Dr. Astrid Nieße, Prof. Dr. Eirini Ntoutsi, Prof. Dr. Ina Schaefer and Prof. Dr. Felipe Temming
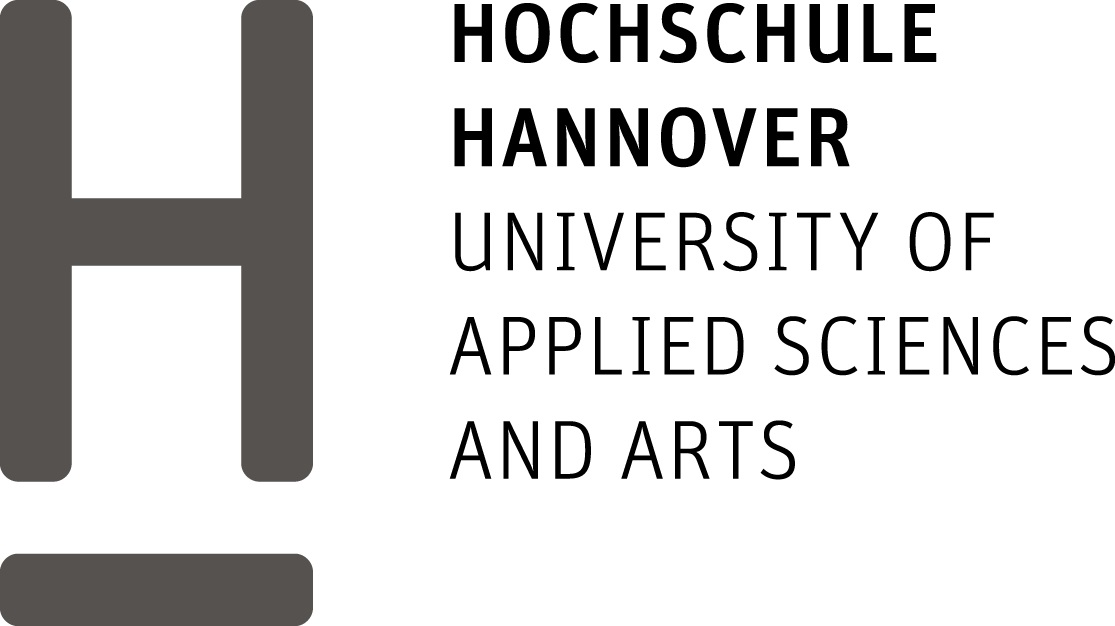
https://www.hs-hannover.de/
Die Hochschule Hannover ist eine Hochschule für angewandte Wissenschaft mit ca. 10.000 Studierenden an fünf Fakultäten. Eingebunden in das Promotionsprogramm sind Hochschullehrer der Fakultät für Medien, Information und Design aus der Abteilung Information und Kommunikation, welche zugleich auch im Forschungscluster Smart Data Analytics der Hochschule Hannover organisiert sind.
Prof. Dr. Ina Blümel, Prof. Dr. Fabian Schmieder and Prof. Dr. Christian Wartena
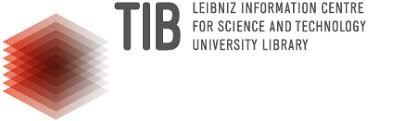
https://tib.eu/
The Leibniz Information Centre for Science and Technology - German National Library of Science and Technology (TIB) is a member of the Leibniz Association and, as the German National Library of Science and Technology, provides science, research and business with literature and information. The TIB conducts applied research and development to generate new, innovative services and optimise existing ones. In addition, the TIB is committed, among other things, to open access and unrestricted access to information, and offers corresponding services and further training.
Prof. Dr. Sören Auer, Prof. Dr. Ina Blümel and Prof. Dr. Ralph Ewerth
Speakers
Researchers
IT-Recht, Datenschutzrecht, IT-Sicherheitsrecht, Urheberrecht